Hey Nick! I’m excited to get started. AI is a hot topic in ad tech, which seems to have replaced machine learning in conversation. Can you clarify the difference between AI and ML?
Yes, I’m happy to. The short version is: ML builds AI. But let me break it down a bit. Drawing from my background in nuclear engineering, it’s like this: nuclear engineering applies an underlying science, physics, to build stuff, like reactors. Similarly, ML applies statistics and computer science, giving us algorithms to build models, some of which are advanced enough to be called AI. So, saying “we do AI, not ML” is like saying “we do planes, not flight.”
Right, right. That makes sense. Knowing AI is a trend in the advertising world right now, I’m wondering what trends you’re monitoring in the data science space?
The revolution being brought about by contemporary AI, such large language models, is that the models are becoming much more general in terms of the coverage of human life and society. Whereas before, text models were very targeted and specific, for example: Is this statement positive or negative? Does this have toxic language? Is it talking about a cat?
Now, you have one model that generally covers everything. On the technical side of it, the model itself contains a lot of general information that can be integrated with other models, which is leading to something called a large multimodal model.
With a large multimodal model, you can have a lot of very different input formats that essentially emulate human sensory data. You can feed it an image or speech patterns, for example, and somewhere inside the model, it can generalize those inputs and merge them into a unified understanding. It’s very exciting to me because the more sensory inputs you integrate, the closer it becomes to being like a person and having an understanding of the data that we as humans interpret innately.
Do you feel the recent spotlight on large language models, like ChatGPT, is drawing attention away from or slowing progress in other fields of ML?
I don’t think so. The machine learning and data industry has gone through a lot of these types of ups and downs where something really hot comes out, some breakthrough happens, and it gets a lot of attention, even if it doesn’t reach mainstream understanding.
Years ago, it was computer vision — where computers can analyze and understand images — and that became the hottest, most prestigious topic in our industry. But across the board, research has chugged along very consistently in every realm, from making really simple models and algorithms more efficient to pushing the boundaries into increasing complexity.
How do you foresee data science and AI evolving over the next 5 to 10 years?
I can see text recognition technology becoming more powerful, hopefully more transparent, and integrating with other categories of models, such as computer vision. Over time, coupling these two categories will make them stronger than they are now. For example, the ability to upload a photo of a basketball game and not only be told what’s contained in the image, but given a very thorough understanding of what’s happening. For example: this basketball player is two seconds away from scoring a three point shot to win a playoff game between team A and team B.
What other future applications have you thought about?
Food delivery. In the future, it might be such that there’s a robot with a small camera and microphone. You could give the robot a sandwich and direct it to go two blocks, make a left, and drop it at a certain door. The robot would understand these directions and have a visual understanding of the environment, enabling them to execute as a human would.
On that note… will the robots take our jobs?
I don’t think so. For the most part, these systems will always require a human with expertise in the loop. As a society, we might get to a point where we’ll trust a robot to do something on a day-to-day basis, but I don’t think we’ll ever get to the point where we’ll rely on robots to diagnose other robots.
People are happy to automate things until they go wrong. And the thing with AI is it’s not like when something breaks down in your car, where the problem is very clear. With these models when something breaks, it’s not obvious. It’ll just give you the highest probability result that it thinks is correct, without outwardly telling you something’s wrong. And someone with expertise will always be required to diagnose it.
On the other hand, organizations that spend billions of dollars to get hundreds or thousands of people to work on one thing may find they can reduce headcount by making a portion of their team more efficient. But for jobs like journalism, for example, you’ll always need people with both a general and deep, technical understanding. We’re not going to let robots grade their own homework.
Follow Nick Tran on LinkedIn and learn more Jun Group’s intelligent advertising solutions for brands and agencies.
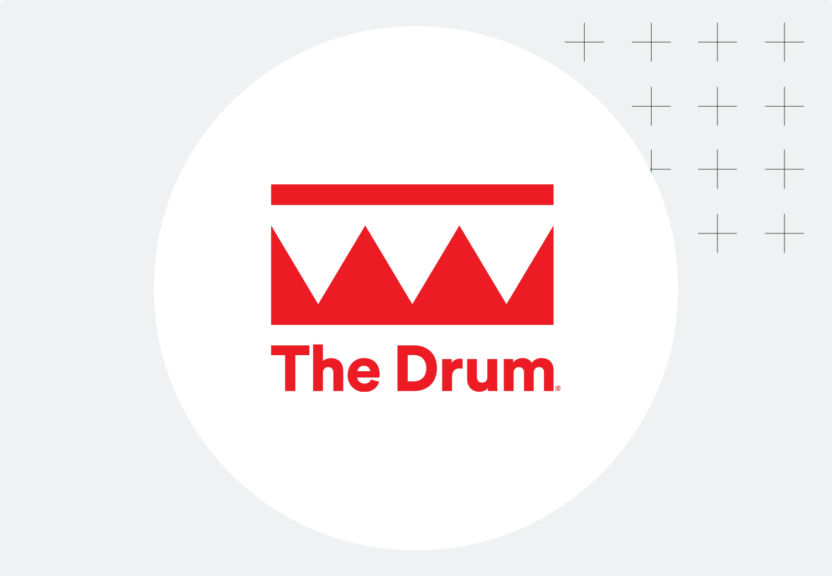
This New Buzzword Will Disrupt Measurement in 2025
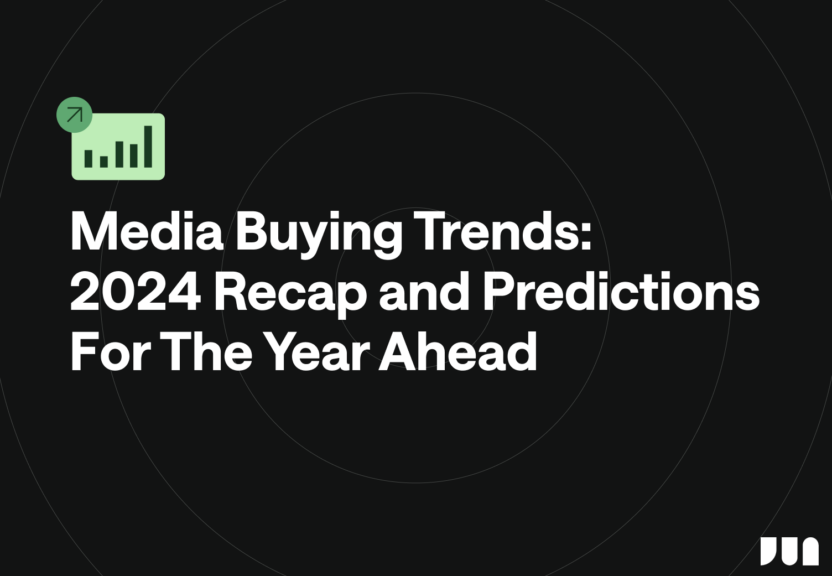
Media Buying Trends: 2024 Recap and Predictions for the Year Ahead
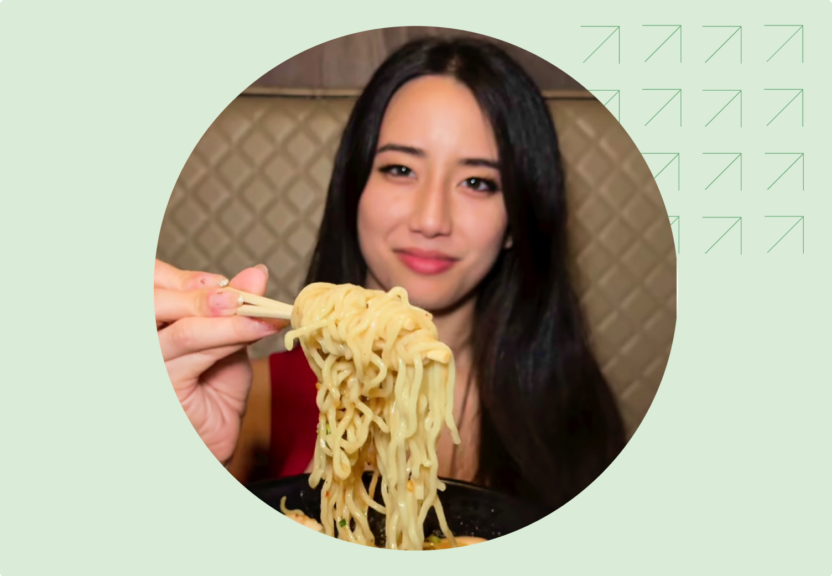